TL;DR: Generative AI Use Cases in Manufacturing (2026 Snapshot)
Generative AI use cases in manufacturing are evolving from experimental to essential. This white paper outlines six high-impact applications already transforming factories, from AI-generated training videos and multilingual safety briefings to simulation-based learning and real-time design feedback loops. It also examines why these shifts are accelerating in 2025, what infrastructure is required to support them, how jobs on the factory floor are being redefined, and the ethical implications of AI-generated content and digital likeness. For manufacturers, this isn’t just new tech. It’s a strategic rethink of how knowledge flows, how training scales, and how decisions are made at speed.
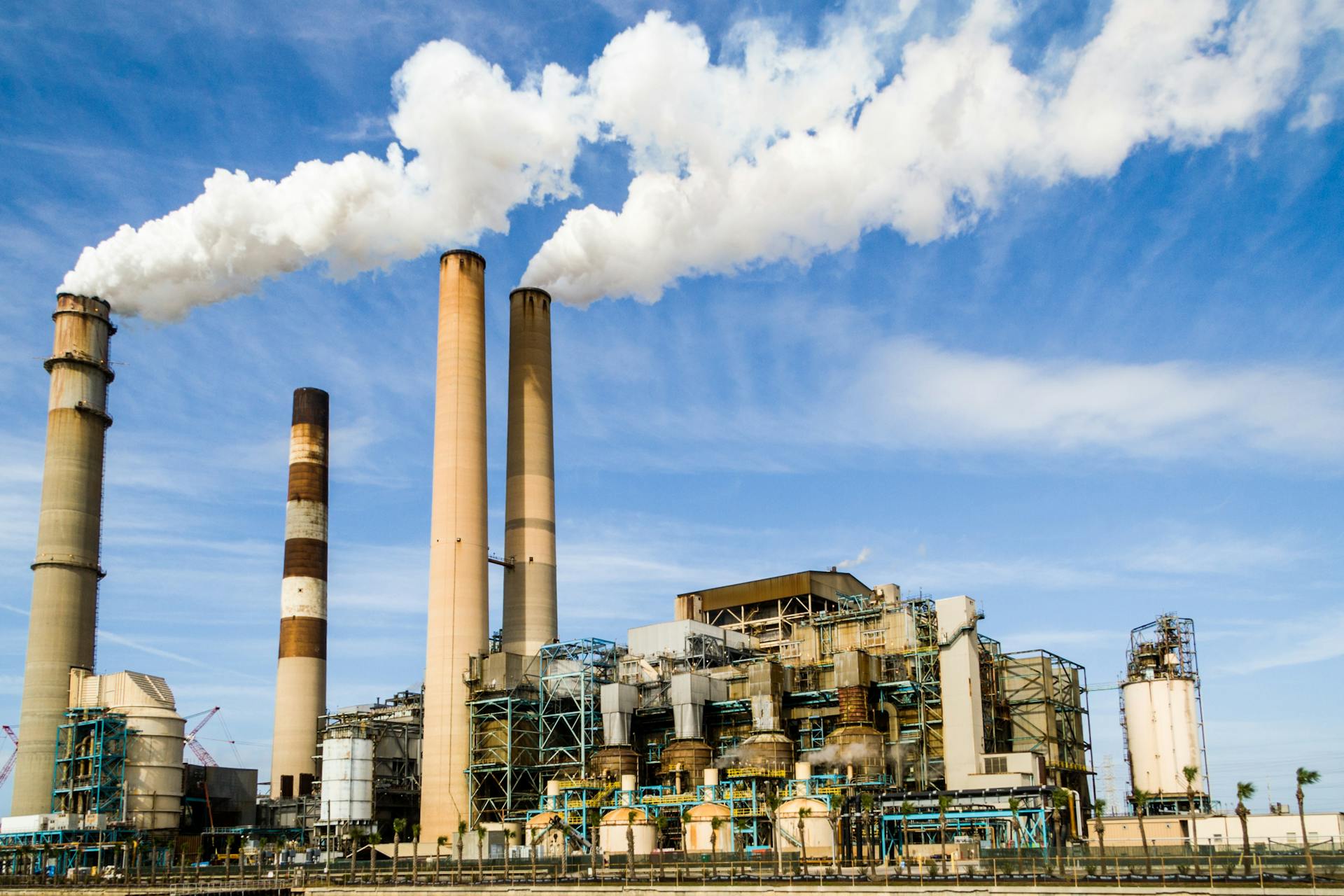
Introduction: A Quiet Revolution on the Factory Floor
Something’s shifting on the factory floor, and it’s not just the machinery. It’s subtler than that. Not a new tool or workflow, but a change in tempo—a different kind of intelligence humming beneath the surface. Not the sort that clanks and sparks, but one that simulates, iterates, and narrates without blinking. The kind that can watch, listen, and... imagine. Today, generative AI use cases in manufacturing have slipped into manufacturing like a new hire with an impressive resume and no clear job description. First welcomed into marketing departments and design studios, it now finds itself writing SOPs, crafting safety briefings, and prototyping next-gen components before human hands ever get involved.
This isn’t automation 2.0. This is a redefinition.
In 2025, factories began to realize that the greatest efficiency didn’t lie in speeding up what was already there, but in questioning it entirely. What if training wasn’t static but evolving in real-time? What if design feedback came in the form of multilingual videos instead of spreadsheets? What if human knowledge could be captured, regenerated, and scaled globally, without diluting its nuance?
This paper explores not just where generative AI is going in manufacturing, but how it’s reshaping what it means to make something. It’s not a technical manual, nor a pitch. It’s a snapshot of a moment, half-built, still forming, on the edge of transformation. Because by 2026, generative AI won’t be the new thing. It will just be... how it’s done.
Data & Industry Insights: Generative AI in Manufacturing by the Numbers
The future rarely announces itself all at once. It leaks in through spending patterns, adoption curves, and the slow drift of priorities. In manufacturing, generative AI didn’t arrive with fanfare. It arrived in budget lines. In timelines that shortened. In charts that suddenly bent upward.
Here’s what the data is showing—quietly, but unmistakably.
In 2024, the generative AI market in manufacturing was valued at $447 million. It grew to $630.72 million in 2025.
By 2034? Projections place it north of $13 billion. That’s not a trend line. That’s a trajectory.
Some project a rise from $223 million in 2023 to over $6.4 billion by 2033, rising at more than 41% CAGR. In other words: steep, sustained, and serious.
Investments Are Following Belief
Manufacturers don’t spend at this scale unless they see something coming. The budgets are shifting, quietly but decisively.
- In 2023, the manufacturing industry invested an estimated $1 billion into generative AI. In 2024, that figure is expected to more than double, approaching $2.4 billion.
- Technology investments now account for 30% of operating budgetsin manufacturing companies. Generative AI isn’t the only reason. But it’s high on the list of reasons why.
Adoption Isn’t Waiting for Perfection
A 2024 snapshot found that 35% of manufacturers were already using AI for tasks like predictive maintenance and quality control.
Another survey suggests that 55% of industrial product manufacturers are now using generative AI in some form and 40% plan to increase investment over the next three years.
This isn’t mass adoption. But it’s not fringe anymore, either. It’s momentum, gathering.
The Friction Points That Still Hold Weight
Not every curve is smooth.
44% of manufacturers say they still don’t fully trust the accuracy of AI responses. Accuracy gaps make adoption harder, especially in safety-critical settings.
Cost and complexity remain top reasons for hesitation. Especially for mid-sized firms without deep internal AI talent.
The opportunity is clear. But the on-ramps still need design work.
Reading the Signal Beneath the Stats
Every number above is a data point. But taken together, they trace something else—a mood. A shift in posture. A sector that isn’t just experimenting anymore, but rethinking.
This isn’t about being early. It’s about being ready.
Because what started as technology is becoming infrastructure.
How Generative AI Is Already Transforming Manufacturing in 2025
It’s easy to mistake this moment for hype. New tools surface weekly, headlines bounce between breakthroughs and disasters, and nearly every software demo ends with “...powered by AI.” But behind the noise, something steady is unfolding in manufacturing. Not spectacle, but systems quietly changing shape.
Generative AI has moved past the pilot phase. It’s being woven into actual workflows.
In training rooms, it’s generating onboarding videos overnight.
In engineering bays, it’s simulating product variations without a single CAD click.
In maintenance logs, it’s drafting service instructions based on real-time data.
But it’s not just adoption that matters, it’s depth. The transition is from reactive to generative, from optimization to collaboration.
Multimodal AI models, those that can process text, video, audio, and 3D data, are making this possible. A training video can be generated from a technical document. A voice-over can be localized into twelve languages in minutes. A failed machine cycle can be reimagined as an interactive learning module by the time the next shift starts.
And importantly, generative AI is not just “thinking”, it’s watching, simulating, and producing. This isn’t about giving machines new tasks. It’s about giving people new tools that learn as fast as the environment changes.
Why Generative AI Use Cases in Manufacturing Are Gaining Momentum
Manufacturing isn’t exactly quick to jump on trends. It values precision, reliability, and repeatability, the kind of legacy thinking that has built airplanes, bridges, and billion-dollar supply chains. So when the sector starts paying real attention to a technology, it’s not because it’s flashy. It’s because something deeper is pushing at the seams.
Generative AI isn’t catching on because it’s clever. It’s catching on because the systems in place are cracking.
1. The Disappearing Workforce
The people who knew the line by heart, the ones who could hear a motor hum and predict a belt slip, are retiring. What they carry in experience is walking out the door, and the replacement pipeline isn’t ready. As per a 2025 report from Deloitte, the global manufacturing skills gap could leave more than 2.1 million jobs unfilled by 2030.
Generative AI doesn’t replace their wisdom. But it helps capture it. Simulate it. Translate it into something teachable.
2. Knowledge That Doesn’t Stay Put
Tribal knowledge is real, and it’s vanishing. Process tweaks scribbled in notebooks. Safety tips passed on verbally. These aren’t in the ERP. Generative AI systems are now being trained to turn tacit expertise into explicit documentation, sometimes overnight.
What used to take a week of interviews and screen captures can now be done in a few minutes.
3. Complexity Across Borders
Global operations bring language barriers, cultural gaps, and inconsistent training standards. A safety protocol that works in English may fall flat in Tagalog or Portuguese. Generative AI doesn’t just translate, it localizes. It adapts. It rephrases and reorders content depending on the audience.
The result is not just understanding. It’s retention.
4. Sustainability Pressure
Factories are being asked to do more with less: less material, less waste, less energy. Generative design tools are helping engineers prototype components that meet performance specs while reducing weight, friction, and environmental impact.
5. From Industry 4.0 to Industry 5.0
The term sounds like a rebrand, but the concept is real. Industry 5.0 isn’t about robots. It’s about resilience. Human-machine collaboration at scale. Generative AI makes this real, not by replacing human judgment, but by supporting it. Suggesting next steps. Simulating failure. Recommending recovery.
The machines are learning to support the humans. And that changes everything.
High-Impact Generative AI Use Cases in Manufacturing
It doesn’t look revolutionary at first. No neon lights. No dramatic announcements. Just a slightly sharper video training module. A safety briefing that feels like it knows who it’s talking to. An engineer who spends less time staring at a blank CAD screen.
This is how generative AI arrives. Not with a parade, but with a whisper that rewrites everything.
Here’s what’s actually happening, behind the scenes, beneath the noise:
1. Training Content That Writes (and Rewrites) Itself
Someone updates an SOP. Ten minutes later, an AI system generates a localized, captioned training video for every site. In English, Hindi, Portuguese. With embedded callouts. With a voice that sounds like a trusted floor manager.
There’s no need to book a trainer. No one digs through SharePoint. New hires don’t learn through guesswork.
And maybe, for the first time, the learning actually sticks.
2. Feedback That Finds Its Way to Design
Engineers used to wait weeks, sometimes months, for customer feedback to reach them. And when it did, it came as a spreadsheet or a terse complaint buried in an email thread.
Now, generative AI stitches together clips from the field, snippets of malfunction, stress points under load, a thousand little signs of wear. It turns them into something watchable. Understandable. Actionable.
Less “what went wrong?”
More “here’s what we can do next.”
3. Safety Briefings That Actually Reach the Worker
A one-size-fits-all safety message doesn’t work when your night shift speaks Hindi, your morning team speaks Spanish, and your warehouse runs 24 hours without pause.
AI generates safety briefings that speak the right language, use the right tone, and show exactly what’s at stake. Sometimes it’s firm. Sometimes it’s gentle. Always, it’s clear.
No more printouts posted near the time clock. Just messages that land.
4. Co-Design That Feels Like Collaboration
There’s a kind of magic in seeing an idea form in real time, especially when that idea talks back.
Engineers are beginning to sketch or describe what they need. And the system listens. It offers five variations, simulates thermal stress, and flags a material shortage in one of them.
This isn’t automation. It’s dialogue.
A kind of quiet collaboration that speeds up thinking without skipping depth.
5. Communication That Cuts Through the Clutter
Internal memos get missed. Dashboards go unread. Shift notes disappear.
Now: an AI avatar delivers a 90-second update, personalized to the team, recapping yesterday and previewing tomorrow. With visuals. With clarity.
It doesn’t just inform. It connects.
Because when the message feels made for you, you’re more likely to hear it.
6. Learning by Failing (Without the Cost of Actual Failure)
Some failures are too rare to practice for, until they happen. A pipe bursts, a bearing slips, a sequence runs backwards. With generative AI, those moments can be simulated, visualized, and walked through before they’re ever lived.
It’s not a theory. It’s muscle memory, built digitally.
Because knowing what to do in the moment shouldn’t depend on having seen it before.
What Powers Generative AI in Manufacturing Behind the Scenes
It’s easy to focus on what generative AI creates—videos, simulations, safety briefings—but the real story lies beneath the surface. Quietly, it’s infrastructure that determines who gets to scale and who gets stuck.
Because this technology doesn’t run on cleverness alone. It runs on structure, on coherence, on systems that know how to listen.
The truth is, most factories weren’t built with this kind of intelligence in mind. Not the digital twins, not the multi-modal models, not the constant exchange between edge devices and cloud platforms. So what’s needed isn’t just adoption. It’s adaptation.
Here’s what that looks like:
Clean, Connected Data
Generative AI doesn’t work with broken inputs. It needs structured logs, interoperable systems, and access to everything from work orders to quality audits. It needs context, not just content.
Spreadsheets in three formats? Not helpful.
Real-time sensor data, tied to versioned designs? That’s the gold.
Data lakes are becoming less of a luxury, more of a baseline.
A Modular Tech Stack
Monolithic ERP systems can’t keep up with real-time creation. Generative AI needs APIs. It needs lightweight services that talk to each other.
Cloud-native platforms enable updates at the pace of need.
AI lets models run where the action happens, in the plant, not the server room.
Hybrid infrastructure means teams don’t have to choose between speed and security.
It’s not about replacing legacy systems. It’s about building connective tissue between them.
Models That Understand the Work
It’s not enough for an AI model to be powerful. It has to be trained on language that sounds like manufacturing.
Not marketing decks. Not casual chat. But terms like torque calibration and bearing misalignment. It needs domain adaptation and contextual awareness that only comes from time spent inside the world it’s serving.
And this is where most off-the-shelf models fall short. They’re generalists. What’s coming next are specialists: industry-tuned, factory-literate, trained on the messy, beautiful edge cases of real-world operations.
Governance That Doesn’t Feel Like an Afterthought
With power comes pressure. As AI systems generate more, more content, more decisions, more visibility, the need for control deepens.
Who signs off on an auto-generated SOP?
What happens when an avatar says something wrong?
How do we trace what data trained the model in the first place?
Audit trails. Human-in-the-loop validation. Ethical review boards. These aren’t legal requirements (yet), but they’re already showing up in the factories that are thinking long-term.
How Generative AI Is Reshaping Jobs in Manufacturing
This part doesn’t make the brochures. There are no glossy press releases for “how a technician feels when their job changes.” But beneath the dashboards and integrations, there’s something human happening. Generative AI isn’t just shifting what factories produce. It’s shifting how people contribute.
And maybe, how they feel about it.
Because when machines can write training manuals, simulate breakdowns, or narrate project updates, the obvious question becomes, what’s left for people to do?
“But the better question is, what can people do now that they couldn’t before?”
From Repeat to Interpret
Repetitive documentation, language translation, formatting, the kinds of things that swallowed time but didn’t require expertise, these are disappearing fast.
In their place?
Technicians curating AI-generated content for clarity and accuracy.
Team leads interpreting AI simulations and coaching others through them.
Operators running what are essentially human-machine feedback loops.
It’s not less work. It’s different work. More reflective. Less reactive.
New Roles Are Emerging
Walk through a forward-looking plant in 2026 and you might hear unfamiliar job titles:
AI workflow designer: someone who maps how generative content flows across teams.
Simulation reviewer: an expert who vets what the AI suggests before it reaches the floor.
Content trust officer: the person who makes sure every auto-generated video still sounds like something the company meant to say.
These aren’t pie-in-the-sky roles. They’re already showing up in aerospace, in automotive, in high-volume consumer goods.
Because when content creation becomes infinite, curation becomes essential.
Reskilling Without the Jargon
There’s a way to talk about upskilling that makes it sound intimidating. Certifications, e-learning platforms, digital badges. But most workers don’t want badges. They want to understand what’s changing, and how to keep doing good work in a system they can trust.
That means:
Learning how to validate AI outputs.
Knowing when to override, when to escalate.
Feeling confident in a world where some tasks have been taken, but new ones are quietly waiting to be picked up.
The factories that will thrive are the ones that don’t just train people to use AI, but invite them to help shape it.
Likeness, Consent, and Control in AI-Generated Manufacturing Systems
There’s something eerie, and oddly intimate, about seeing yourself in a video you didn’t film. About hearing your voice say something you never said. In manufacturing, this isn’t a distant dilemma. It’s already here.
A supervisor’s face animates a safety demo. A technician’s voice explains a process step-by-step. A frontline worker appears in onboarding videos for a facility halfway across the world.
None of it was manually recorded.
All of it was generated.
And it raises a question that’s less about technology, and more about identity.
Who Owns the Digital Twin?
A digital likeness can be trained on just a few minutes of footage. Once it exists, it can narrate, instruct, even react. But:
Does the individual control how that likeness is used?
Can they revoke it?
Should they be compensated if it’s deployed at scale?
In Europe, the AI Act enforces consent, transparency, and opt-outs for synthetic media. But most global frameworks are still catching up. Meanwhile, the tech races forward.
Ethics here isn’t just compliance. It’s a cultural choice. Some are already leading with that mindset. Platforms like Personate AI embed ethical checks into every layer—from how content is screened to how consent is managed. Personate AI’s systems are fully GDPR-compliant and built with transparency at their core.
When “Close Enough” Isn’t Good Enough
Generative content can be startlingly accurate, until it’s not. A missed instruction. A mispronounced phrase. An oversimplified task.
These aren’t bugs. They’re design choices. Because generative AI doesn’t just reflect reality. It reconstructs it. And when the stakes involve worker safety or equipment reliability, “almost right” can be dangerous.
That’s why human review isn’t a box to check. It’s a system to build.
Trust Is the Foundation, Not the Output
There’s a temptation to measure success in speed. How many hours saved. How many videos generated. But in manufacturing, speed means nothing without trust.
Trust that the AI isn’t hallucinating.
Trust that what was said reflects what was meant.
Trust that workers aren’t being surveilled, replicated, or erased.
Factories are not labs. They’re places where people show up every day, do hard work, and rely on each other to stay safe. Generative AI can be a partner in that. But only if it’s designed to earn its place.
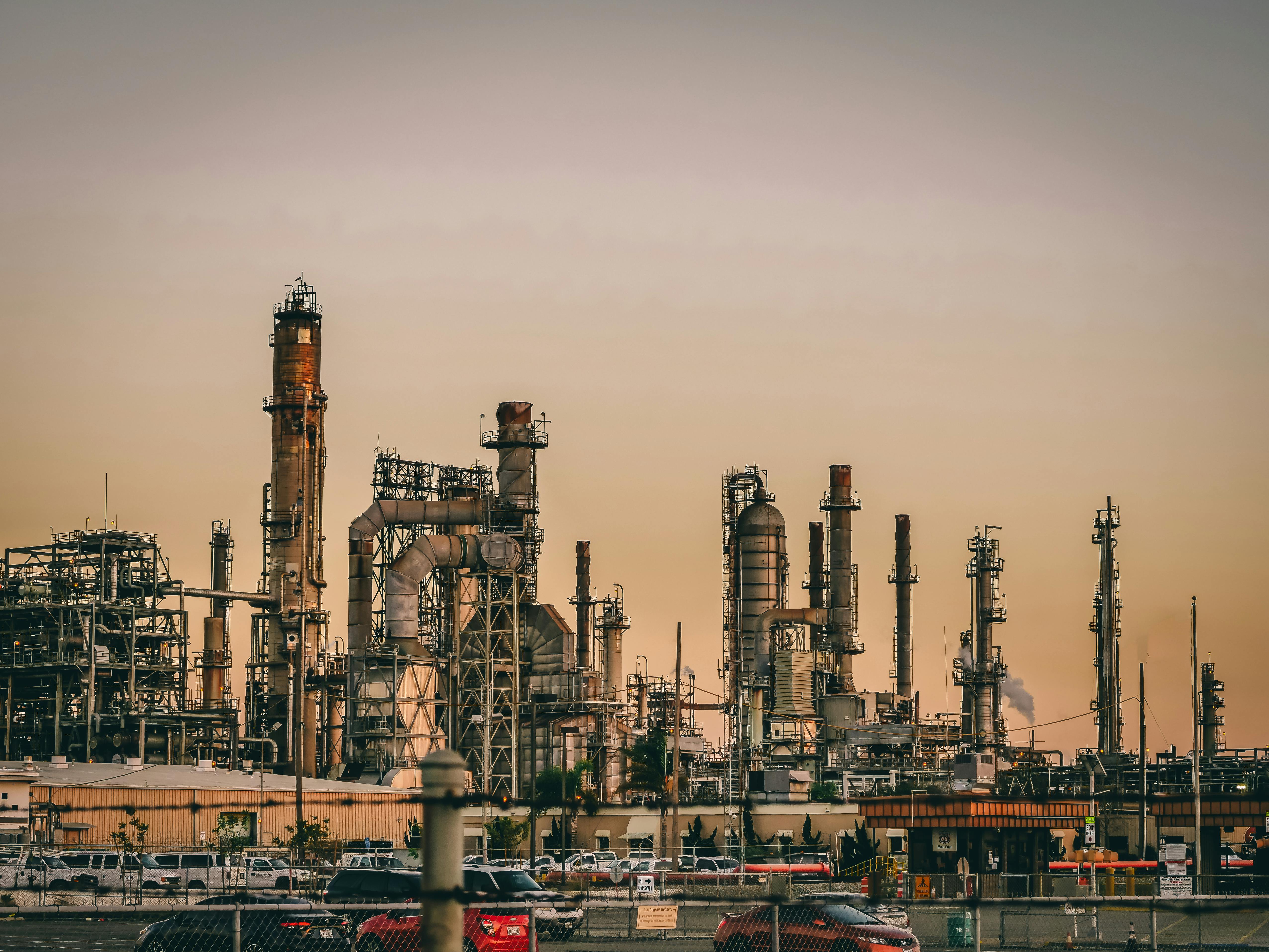
What Manufacturing Leaders Must Do Now to Stay Ahead
There’s a moment, just before change becomes irreversible, when leaders have a chance to shape its direction. This is that moment for manufacturing. Not because generative AI is the newest tool, but because it might be the first one that invites the industry to rethink its foundations.
This isn’t about buying software. It’s about rebuilding mental models.
The leaders who will define the next decade aren’t just asking what generative AI can do. They’re asking what they want it to mean.
Questions worth sitting with:
Are we building infrastructure that invites evolution—or patches over dysfunction?
Can our people trust the systems we’re asking them to rely on?
What does excellence look like when knowledge can be automated—but wisdom cannot?
Who decides how our digital likenesses are used? And when?
These aren’t questions with quick answers. They require discussion. Debate. Deep listening.
But the companies that start now, who treat generative AI not as a feature but a shift, will move differently.
They’ll build:
Feedback systems that adapt in real time.
Training ecosystems that evolve alongside operations.
Communication flows that connect people, not just departments.
A culture that sees AI not as a threat, but as a chance to grow sharper, faster, more human.
The Future of Generative AI in Manufacturing Isn’t Promised. It’s Built.
The future isn’t being delivered. It’s being built. Every process redesigned, every policy rewritten, every video generated, it’s all part of the scaffolding for what comes next.
And the factories that thrive won’t be the ones with the flashiest demos. They’ll be the ones that ask better questions. They’ll be the ones that know how to listen.
Where to Go From Here
Generative AI in manufacturing isn’t just an upgrade, it’s a shift in what’s possible. The leaders who succeed won’t be the ones with the most tools, but the ones who ask better questions and build smarter systems.
If you’re ready to take the next step, from experimentation to implementation, Personate AI and its sister company AiReel.io are ready to help.
Personate AI specializes in generative video infrastructure for global teams—turning SOPs, training docs, and field insights into high-impact, multilingual content.
AiReel.io lets you turn product pages and technical URLs into dynamic explainers in minutes—helping engineers, buyers, and operators stay aligned, informed, and engaged.
Both are built for speed, scale, and integrity. GDPR-compliant. Ethically grounded. Designed to help manufacturers move fast without breaking trust.
Have a use case? A challenge? A question?
📬 Reach us anytime at hello@personate.ai or team@aireel.io. Let’s explore how AI can work where it matters most—on the floor, in the field, at the front line.